First the definition from Wolfram
A Markov chain is collection of random variables {X_t} (where the index t runs through 0, 1, …) having the property that, given the present, the future is conditionally independent of the past.
Wikipedia is a little clearer
…Markov chain is a stochastic process with markov property … [Which means] state changes are probabilistic, and future state depend on current state only.
Markov chains have various uses, but now let’s see how it can be used to generate gibberish, which might look legit.
The algorithm is,
- Have a text which will serve as the corpus from which we choose the next transitions.
- Start with two consecutive words from the text. The last two words constitute the present state.
- Generating next word is the markov transition. To generate the next word, look in the corpus, and find which words are present after the given two words. Choose one of them randomly.
- Repeat 2, until text of required size is generated.
The code for this is
To see a sample output, we take the text of My man jeeves by Wodehouse from Project Gutenberg, and see a sample output.
In [1]: file_ = open('/home/shabda/jeeves.txt')
In [2]: import markovgen
In [3]: markov = markovgen.Markov(file_)
In [4]: markov.generate_markov_text()
Out[4]: 'Can you put a few years of your twin-brother Alfred,
who was apt to rally round a bit. I should strongly advocate
the blue with milk'
[The files you need to run this are jeeves.txt and markovgen.py]
How is this a markov algorithm?
- The last two words are the current state.
- Next word depends on last two words only, or on present state only.
- The next word is randomly chosen from a statistical model of the corpus.
Here is some sample text.
“The quick brown fox jumps over the brown fox who is slow jumps over the brown fox who is dead.”
This gives us a corpus like,
{('The', 'quick'): ['brown'],
('brown', 'fox'): ['jumps', 'who', 'who'],
('fox', 'jumps'): ['over'],
('fox', 'who'): ['is', 'is'],
('is', 'slow'): ['jumps'],
('jumps', 'over'): ['the', 'the'],
('over', 'the'): ['brown', 'brown'],
('quick', 'brown'): ['fox'],
('slow', 'jumps'): ['over'],
('the', 'brown'): ['fox', 'fox'],
('who', 'is'): ['slow', 'dead.']}
Now if we start with “brown fox”, the next word can be “jumps” or “who”. If we choose “jumps”, then the current state is “fox jumps” and next word is over, and so on.
Hints
- The larger text we choose, the more choices are there at each transition, and a better looking text is generated.
- The state can be set to depend on one word, two words, or any number of words. As the number of words in each state increases, the generated text becomes less random.
- Don’t strip the punctuations etc. They lead to a more representative corpus, and a better random text.
Resources
Did you know that markov chains have many other uses in finance, statistics and mathematics? In fact Google’s page rank algorithm is essentially a markov chain of the web! We publish articles on Algorithms, Python, Django and related technologies every week. Why not Subscribe to us or follow us on twitter?
Thank you for reading the Agiliq blog. This article was written by shabda on Jun 16, 2009 in algorithms, , python .
You can subscribe ⚛ to our blog.
We love building amazing apps for web and mobile for our clients. If you are looking for development help, contact us today ✉.
Would you like to download 10+ free Django and Python books? Get them here
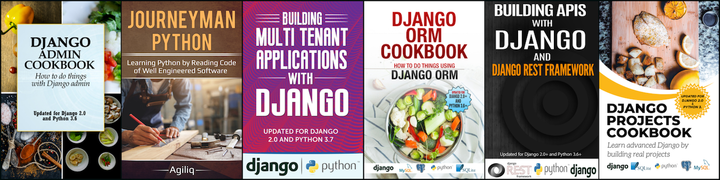